Exploring Machine Learning Approaches for Time Series: A Bibliometric Analysis
DOI:
https://doi.org/10.5530/jcitation.1.1.6Keywords:
Machine Learning for Time Series, Bibliometrics, Forecasting, Topic analysis, CollaborationAbstract
Our paper analyzes 20 years of Machine Learning for Time Series forecasting research published in journals, books, papers. We analyzed the bibliographic collections and bibliographic services present on Scopus, the largest database of abstracts, citations of literature and quality web sources, which includes scientific journals, books and conferences, extrapolating the quantitative relationships between documents and their elements. Through this analysis, we analyzed the main information on the structure of the data, such as total citation by country, the documents with the highest number of citations, the most productive authors, the most important keywords. We also obtained graphs of the most productive authors, the average total citations per year, the annual scientific production and the average number of citations of the article per year, as well as an evolution of the topics and a thematic map.
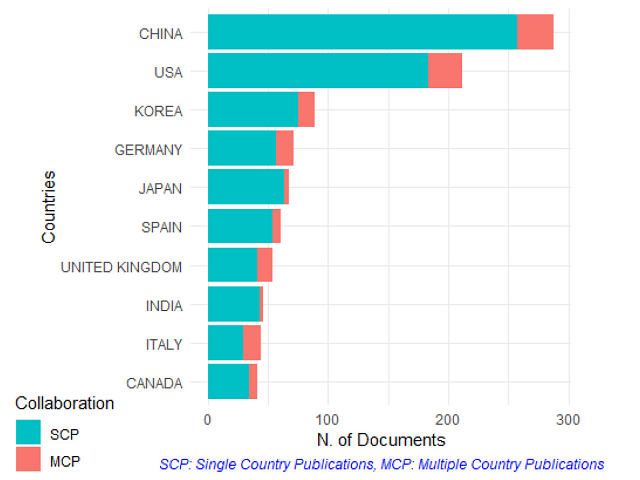
Downloads
Published
How to Cite
Issue
Section
License
Copyright (c) 2022 The Author(s)

This work is licensed under a Creative Commons Attribution-NonCommercial-NoDerivatives 4.0 International License.